Introduction:
In the ever-evolving landscape of technology and data, the role of a data scientist has become increasingly pivotal. As organizations harness the potential of big data, artificial intelligence, and machine learning, the demand for skilled data scientists is at an all-time high. One key attribute that distinguishes a proficient data scientist from the rest is their Quantitative Quotient (QQ). In this comprehensive article, we will explore the significance of QQ in data science, its components, and strategies to elevate these skills to meet the demands of the dynamic industry.
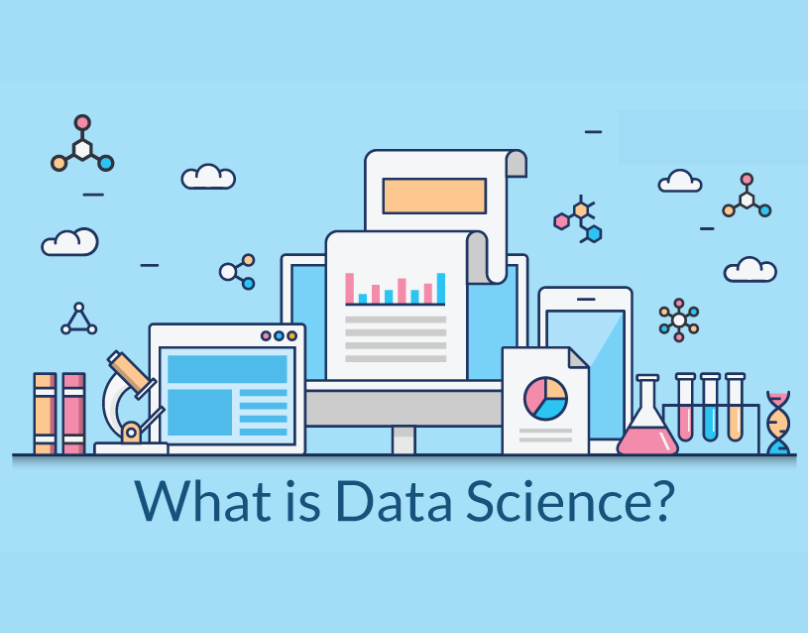
Understanding Quantitative Quotient (QQ):
Quantitative Quotient refers to the ability to understand, analyze, and interpret quantitative data effectively. It encompasses a range of skills, including statistical analysis, mathematical modeling, and data visualization. A high QQ empowers data scientists to make informed decisions, identify patterns, and extract meaningful insights from complex datasets.
Components of Quantitative Quotient:
Statistical Proficiency:
A strong foundation in statistics is the cornerstone of a high QQ. Data scientists should be adept at applying statistical methods to draw valid conclusions from data. This includes understanding probability, hypothesis testing, and regression analysis.
Mathematical Modeling:
Data science often involves creating mathematical models to represent real-world phenomena. A high QQ involves the ability to develop, validate, and interpret these models accurately. Proficiency in linear algebra, calculus, and optimization techniques is crucial.
Data Visualization Skills:
Communicating insights effectively is a vital aspect of data science. Data scientists with a high QQ can create compelling visualizations that simplify complex information. Mastery of tools like Tableau, Matplotlib, or Seaborn is essential.
Strategies to Elevate Quantitative Quotient:
Continuous Learning:
The field of data science is dynamic, with new techniques and tools emerging regularly. Data scientists should engage in continuous learning through online courses, workshops, and reading relevant literature to stay abreast of the latest trends.
Practical Application:
Theoretical knowledge is valuable, but applying it in real-world scenarios is crucial. Engaging in hands-on projects, participating in hackathons, and collaborating on open-source initiatives can provide practical experience to enhance QQ.
Mentorship and Networking:
Learning from experienced professionals can significantly accelerate skill development. Seek mentorship from seasoned data scientists, participate in industry conferences, and join online communities to expand your network and gain insights into best practices.
Diversify Learning Resources:
Explore a variety of learning resources, including books, online courses, tutorials, and research papers. Diversifying your sources of knowledge ensures a well-rounded understanding of quantitative concepts.
Build a Strong Foundation:
Ensure a solid understanding of foundational concepts in mathematics and statistics. Strengthening the basics provides a stable platform for tackling advanced data science challenges.
The Role of Quantitative Quotient in Career Advancement:
A high Quantitative Quotient not only makes a data scientist more proficient in their current role but also opens doors to career advancement. Employers actively seek candidates with strong QQ as it indicates the ability to handle complex data challenges and contribute to strategic decision-making.
Conclusion:
In the rapidly evolving field of data science, a high Quantitative Quotient is the key to unlocking new opportunities and staying ahead of the curve. By focusing on continuous learning, practical application, mentorship, and building a strong foundation, aspiring and experienced data scientists alike can elevate their QQ and make a significant impact in the world of data. As the demand for skilled professionals continues to rise, investing in the development of quantitative skills is a strategic move that pays dividends throughout a data scientist's career. For those seeking the best Data Science Training Course in Agra, Bhopal, Noida, Delhi and all cities in India this commitment to enhancing Quantitative Quotient becomes even more critical. Choosing a comprehensive training program that emphasizes practical skills, real-world applications, and mentorship can be the catalyst for a successful and rewarding journey in the dynamic field of data science.
留言